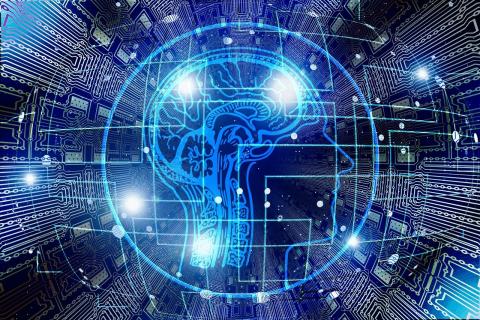
The BioLaw Journal published its last issue of the year (4/2021).
It contains a permanent section: “artificial intelligence”, which deals, in an interdisciplinary and comparative perspective, with the two-way relations that AI generates in contact with the law (impact of law on AI and impact of AI on law).
Following the aim to combine theoretical analysis with practical examples (in order to effectively convey the characteristic features of the relationship between this technology and the legal phenomenon) this issue includes two reports presenting the results of a survey dedicated to how some Italian administrations are facing the challenges of AI are disseminated.
The reports published (edited by Edoardo Chiti, Barbara Marchetti, Nicoletta Rangone) are based on two study seminars dedicated to an empirical investigation on the use of artificial intelligence in public decision-making processes of rule-making, adjudication and enforcement. The first event, entitled “The use of artificial intelligence in the activities of the Bank of Italy”, was held on Wednesday, 28 April 2021. The second seminar focused on the experience of AGCOM, ARERA and CONSOB took place on 25 May 2021. Both the events were organized by the Lumsa University, the University of Trento, the University of Tuscia, the Scuola Superiore Sant'Anna di Pisa, and the ASTRID working group on "Administration and Artificial Intelligence", an expression of the Digital-LED Ecosystem Laboratory.
By taking into account the incomplete and uncertain nature of the existing regulatory framework, the reports offer a significant mapping of the AI applications used (or being tested) and their potential in relation to both the different public functions exercised and the different administrations involved. What emerges is a picture that makes it possible to effectively reconstruct the advantages and limits, potential and risks of the use of AI in the public sphere.
The questions that were discussed with the officials of the various administrations involved were varied and included the following:
- What is the body that takes decisions on the advisability of using artificial intelligence mechanisms? By what means is this (if any) information given to the outside world? Are decisions made as part of the internal design process, or do they also respond to external inputs (e.g., from research or the market)?
- What types of AI systems are actually used? And for what purposes? For example, are artificial intelligence systems used in the pre-trial phase of the process or in the decision-making phase? Are there examples of use that are considered particularly significant?
- What procedure is followed to acquire AI systems? From what sources is it governed? How is it articulated?
- Is the construction of AI systems left to the technicians or is the process managed by the au-tority? What data, information, and requirements are passed on to the engineers to build the AI system? What, if any, are the ways in which interaction takes place between the authority and the technicians called upon to develop the artificial intelligence systems? Is there a validation/certification of the artificial intelligence system? What relationships are established with the provider? What is the du-rate of the contracts?
The aim was not to present the results of the reflection in the traditional ways of research, but rather to make available to interested readers a summary of the reasoning that developed in the conversation among the participants, the picture that emerged, and the problems that were identified.
From the seminar involving Consob, Agcom and ARERA, it has emerged that Consob has opted for an organizational model that allows for a systematic approach to innovation, which looks at the evolution of the markets, both to provide an adequate supervisory response (digital innovation incubator), and to support innovation in the markets (sandboxes and fintech). The experiments underway on the supervisory side concern, with regard to financial products, controls on KIDs and PRIIPs based on risk and machine learning and, with regard to market abuse, intelligent crowling to identify online platforms through which confidential activities are abusively carried out.
Of particular interest is also the activity carried out by the Authority for Communications Guarantees to detect online disinformation through systematic monitoring of newspaper articles and websites, transcripts of television and radio broadcasts, tweets/posts published on platforms, the results of which are published by the Observatory on online disinformation specifically set up. Finally, ARERA's experience is varied. Although it does not make use of artificial intelligence systems in the strict sense of the term, it has highlighted three progressive levels of use of intelligence in data analysis.
A full version of the report describing the experiences and the emerging evidences and criticalities is available here.
From the seminar involving the Bank of Italy, the report resulted in analyzing:
- the AI and perspectives of computer architecture evolution. Concerning the experience of the Bank of Italy, it is represented as the IT Department embarked on a path to organically address this technological domain, noting that the drivers of change described above are rapidly materializing. This path makes use of a central element called the "operational framework for artificial intelligence/machine learning": this is an IT architecture defined by the Informatics Department that combines aspects of an operational nature, as its very name indicates, but also involves elements of method and governance.
- The Big Data, Machine Learning and Artificial Intelligence in economic and statistical analysis, in which it is represented as the research areas on which Bank of Italy focused its attention are the following: i) applications of textual analysis and NLP; ii) applications of machine learning algorithms and neural networks; iii) applications using data from the web or transactional data, such as, for example, credit card transaction data.
- Artificial intelligence and banking and financial supervision, by presenting two examples relating to the two souls of the activity of prudential supervision, aimed at preserving the sound and prudent management of the supervised subjects: the first is the inspection, the second is the remote one. Concerning the inspections, the potential success of the experimentation presented could not only facilitate the preparation and review of an inspection report but also further improve the consistency of treatment. Concerning remote ones, the Bank of Italy is experimenting with the application of techniques of named entity recognition, to identify specific information (entities) in the texts, and of sentiment discovery, to try to derive an informative signal from the business documentation. The Bank of Italy has also conducted experiments in the area of protection supervision, by the Protection and Financial Education Department. These have involved the application of text mining and machine learning techniques to the analysis of a particular category of texts: the complaints that bank customers submit to the Bank of Italy to report potentially unfair practices by intermediaries and alleged violations of transparency regulations.
- Financial intelligence: three examples of application The first, ongoing and potentially one of the most important, is a machine learning project for the automatic classification of STRs. The second project is eminently statistical: using self-constructed bank indicators of money laundering risk, aimed at measuring the exposure to money laundering risk of individual banking intermediaries that can thus contribute to inspection planning. Presented as a useful tool given the limited resources typically available to authorities to inspect many entities. The last project is that of a mafia infiltration risk indicator for firms, based on balance sheet data, thus on public data of firms. It was initially developed with econometric methods, but the Unit is now working on a possible evolution with machine learning techniques.
A full version of the report describing the experiences and the emerging evidences and criticalities is available here.
The next issue of the Review (1/2022) will be containing two additional reports dedicated respectively to Smart Cities and Central State Administrations, in the same section dedicated to Artificial Intelligence.