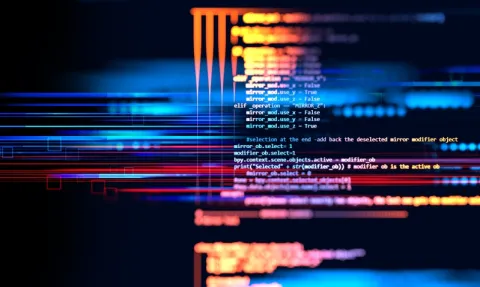
Original article here
With a federal regulatory code running thousands of pages long, how do regulatory agencies find the time to review and update the rules that shape everyday behavior?
One solution might be artificial intelligence (AI)—algorithms that can learn and problem solve in place of humans. But using AI tools to update regulations may compromise valuable transparency and accountability ideals in government, according to an article by New York University School of Law professor Catherine M. Sharkey.
Typically, when agencies need to review and update their rules, human officials make the decisions. Using the “old-fashioned” approach for rule review, officials consider when the rule was issued, if it had been modified, how long ago it had been reviewed, and whether it should be updated to reflect developing technologies.
The Obama Administration, for example, took the old-fashioned approach and asked officials to personally flag potential rules for review, prioritize rule updates, and examine public comments about the rules. In addition, Obama-era officials were required to submit a report detailing their review process and findings.
The Trump Administration chose a different path. With a focus on deregulation, the Trump Administration required that agencies eliminate two regulations for every one regulation issued. Moreover, the Trump Administration created task forces to identify regulations that impede job creation or are unnecessarily burdensome.
Although the Trump Administration focused on deregulation—unlike the Obama Administration—both administrations used the old-fashioned approach. But this changed in 2021, when the U.S. Department of Health and Human Services (HHS) issued a final rule that incorporated AI into its rule review process.
HHS was the first agency to pilot an AI system that flags outdated, redundant, ineffective, or overly complex or burdensome rules in the review process. Using the system, HHS found that its regulations adopted prior to 1990 had never been edited, and 300 citations contained in these regulations were broken. It also identified more than 50 instances of overlaps in obligations—placing extra burdens on hospitals and healthcare providers.
Although some scholars and agency employees praise the use of AI in administrative matters, Sharkey contends that HHS did not sufficiently describe how AI was used in the review process. Sharkey also maintains that HHS should have disclosed how its AI tools function and when exactly AI was being used in the review process.
Furthermore, Sharkey expresses concern that HHS was not required to notify the public about its AI review process. Under the Administrative Procedure Act, rules that substantively impact regulated communities must undergo a process of public notice and solicitation of comments.
Sharkey and other scholars clarify that AI is only subject to the notice-and-comment process if an agency predominantly relies on AI to make a decision, but not if the agency merely considers AI information in making its final decision. HHS explained that its AI review process made only cosmetic and organizational changes to regulations—for example, correcting “references to other regulations, misspellings and other typographical errors.” Sharkey, however, argues that even these non-substantive modifications deserve more notice and public input, as they may lead to more tangible rule changes in the future.
Sharkey also cautions that an agency might use AI in a partisan or other undesirable way. For instance, an administration could program AI to target specific industries in the review process, leading to deregulation of select industries based on the policy preferences of the current administration.
To address transparency and accountability concerns, agencies should disclose when, how, and why AI is being used in the review process, argues Sharkey. She explains that such disclosure will build trust, facilitate public scrutiny of rules, and help technical experts understand how AI makes its decisions in the rule review process.
In addition, Sharkey urges agencies to seek public input and comments before even using AI to review its rules. Ideally, AI should undergo good old-fashioned notice and comment just like other agency rules.
A court would tend to agree, according to Sharkey. Sharkey predicts that courts will require agencies to disclose information about AI, such as how AI algorithms operate and AI’s accuracy in flagging rules suitable for review.
But even if agencies disclose information about their AI tools, will the public be able to understand how such tools work?
Sharkey concedes that human understanding of AI systems is not always perfect and that technical challenges in writing an AI algorithm make it difficult to communicate AI’s capabilities transparently. She reasons further that disclosure and transparency are not static ideals. Rather, the depth of needed disclosure will depend on the rule’s subject matter and importance.
But too much disclosure could bring its own problems. Sharkey suggests that stringent AI disclosure requirements may cause officials to avoid using AI altogether, or officials might only disclose information in specific situations.
As the world becomes more controlled by robots and other AI systems, Sharkey’s warnings will only grow in salience. She warns that society must not compromise its commitment to democratic values of transparency and accountability. Requiring disclosure of AI’s programing and the factors affecting its rule review process may be the best pathway to reap the benefits of AI without significantly risking democratic values, she concludes.
Omar Khodor
Submitted on Mon, 12/20/2021 - 20:21